
The prospect of a higher-yielding Corn Belt could rest – or advance – on a conveyer belt monitored by cameras that boast superhuman sight, according to new research from the University of Nebraska-Lincoln.
Known as a high-throughput phenotyping system, the automated set-up resides at the Greenhouse Innovation Center on Nebraska Innovation Campus. The system can rapidly measure and compare the physical traits, or phenotypes, of different crop varieties by transporting plants through several 360-degree imaging chambers.
Researchers Yufeng Ge and James Schnable are investigating how the phenotyping system, one of just a few in the United States, can be used to estimate certain properties of corn. The crop’s unwieldy size and complex anatomy have left it mostly ignored by previous automated phenotyping work, the researchers said.
In a recent study, Ge and Schnable demonstrated that images taken by the system’s hyperspectral camera – a technology that detects a much wider range of the electromagnetic spectrum than the human eye – can help quantify the amount of water in a corn plant. Whereas a conventional camera detects wavelengths of only visible light, the system’s hyperspectral camera can capture 240 slivers of wavelengths from both the visible and near-infrared portions of the spectrum.
“In a lot of previous studies, phenotyping was just trying to quantify the size and growth of the plants,” said Ge, assistant professor of biological systems engineering. “But we were also trying to answer the question of whether we can use a hyperspectral imaging system to predict water content, which is one of the most important (traits) for plant physiology and breeding. We were fairly successful in doing that.”
The researchers already knew that water-filled plant tissues absorb different wavelengths of light – and absorb the same wavelengths differently – than do their drier counterparts. Using this knowledge, they applied statistical methods to connect changes in various wavelengths with known changes in the water content of corn plants. This allowed them to build a mathematical model that hewed closely to measurements of actual water content, the study reported.
“This is essentially the same technology we use to analyze the atmospheres of planets in other solar systems,” said Schnable, assistant professor of agronomy and horticulture. “You know the intensity of all the light coming from the star, so when a planet comes in front of the star, you look at what wavelengths (disappear). Similarly, we know the wavelengths of all the lights pointing at the plant, so we can look at which ones come back from the plant and which ones don’t. That lets us see what’s (being absorbed).
“The exciting thing here is that there are now so many things that we could potentially measure. So we have this whole new challenge. What are the measurements that are going to be the most informative? We don’t even know in a lot of cases. Before automated phenotyping technology, we picked the measurement that was easy to make. Now there are thousands of measurements that are, in principle, equally easy to make. It’s a very good problem to have.”
Second sight
Ge and Schnable also showed that conventional RGB imagery from the phenotyping system can be used to estimate the daily growth of corn plants – and how efficiently they use water to stimulate that growth – during their first few weeks of development.
“There are probably other studies that have looked at corn seedlings,” Schnable said. “But I don’t think anyone has been able to take corn to the advanced stage of development while doing this type of imaging because it’s a big plant that wouldn’t fit into the smaller imaging chambers used in other automated phenotyping systems.”
The researchers began by feeding daily images of each plant from two perpendicular angles into a program capable of distinguishing plant from background. Mathematical software averaged the two images into one, approximating a plant’s total surface area by counting the number of plant-covered pixels in the composite image.
Ge and Schnable found that the software’s estimates of plant size correlated strongly with their own measurements of plant weight, leaf area and water use efficiency. The methods required to establish those baseline values help illustrate why the RGB and hyperspectral imaging techniques should prove so useful, the researchers said.
The phenotyping system does automatically weigh and water the plants at regular intervals, allowing the team to periodically measure water consumption of sampled plants. But teasing apart a plant’s water weight from its new biomass growth – and subsequently determining how efficiently each plant turned water into new tissue – required multiple steps that ultimately destroyed the plant. The researchers previously had to remove a plant from soil, weigh it, then dehydrate it in an oven before weighing it again. They also employed a scanning instrument to individually measure the surface area of leaves, a step that required cutting each leaf off the plant.
In killing the plant, these methods kept the researchers from observing how it would have grown and developed afterward. Hyperspectral and RGB imaging not only address this issue, Ge said, but should also further speed the process of simultaneously comparing multiple traits among plant varieties.
“How to capture all of these dynamic traits is really a challenging task without these high-throughput systems,” Ge said. “Now we can take daily images and put them together. We can analyze the growth rate and look at the changes over time at different developmental stages. I think that’s the beauty of this phenotyping that wasn’t really possible in the past.”
Greenhouse to green acres
The team is also working with colleagues from the Department of Computer Science and Engineering to refine its image-analysis program. The hope is that it can eventually distinguish among components of a corn plant – individual leaves, stem segments, ears and more. Achieving that level of specificity might allow it to recognize and track those same components across changes in appearance and location, an important consideration for a plant that develops as quickly and dynamically as corn.
And in an effort to ensure their work will be useful to farmers, Ge and Schnable recently finished growing 140 corn hybrids from major seed companies in both the greenhouse and a research field that simulates the agricultural conditions of Nebraska farms. The researchers are currently analyzing their greenhouse data and comparing it with that from the field, aiming to glean insights on how well the former translates to the latter.
“That’s a very important connection we need to make,” Schnable said. “We don’t want to just figure out how plants grow in a greenhouse. This data has to be relevant to field conditions. Hopefully we can build that into our models to some extent, so that we can make testable predictions in the greenhouse about what’s going to happen in the field.”
Ge and Schnable reported their recent findings in the journal Computers and Electronics in Agriculture. They authored the study with Geng “Frank” Bai, a postdoctoral researcher in biological systems engineering, and Vincent Stoerger, the plant phenotyping facilities manager at the Greenhouse Innovation Center.
The researchers received support from the Agricultural Research Division, housed within the university’s Institute of Agriculture and Natural Resources.

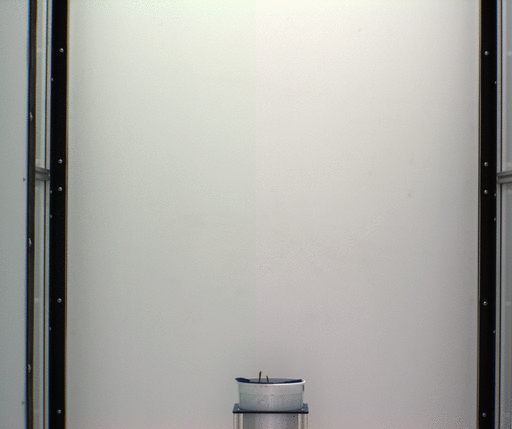
