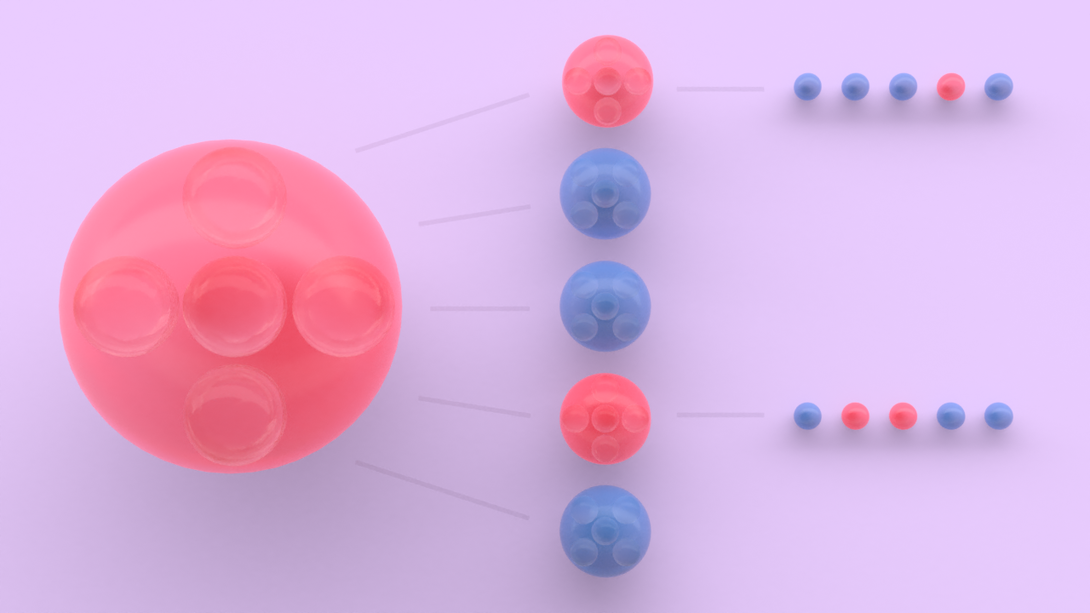
Social distancing. Self-quarantining. Alone, together.
Absent a vaccine, spreading out has become the greatest deterrent to spreading the novel coronavirus, which has now been confirmed in more than 775,000 Americans and 2.4 million people worldwide.
The fastest path back to normalcy, meanwhile, rests on mass-testing for the virus: who has it, who hasn’t, who did but now doesn’t. And the fastest way to determine that, says the University of Nebraska–Lincoln’s Chris Bilder, is actually by grouping — not people, but samples.
Since mid-March, the professor of statistics has advised the Nebraska Public Health Laboratory on its use of group testing, an approach that can save time and resources by reducing the number of tests needed to diagnose infections.
Group testing is an intuitive, one-bad-apple approach to sussing out positive cases. Instead of testing an individual sample from each person, the simplest form involves grouping multiple samples into a pool, then testing that entire pool for the virus or bacterium of interest.
If a pool of 25 samples were to come back negative, all 25 people could be declared infection-free, reducing the overall number of tests by 24. If that pool instead tested positive, a clinic could separate it into smaller pools — five pools of five samples, for instance — and retest. And if, say, two of those smaller pools turned up positive, the clinic could then individually test the remaining 10 samples. Even in the latter scenario, a clinic would save nine of the original 25 tests.
The Nebraska Public Health Laboratory currently starts with a pool of five samples, testing each sample only if the pool comes back positive for the novel coronavirus. In an April 9 paper published by the Royal Statistical Society and the American Statistical Association, Bilder and his colleagues reported that the lab spent 58% fewer tests over its first six days of pooling than it would have by testing just individual samples. That, in turn, meant the lab managed to test 137% more people than it could have using the same resources to test individual samples alone.
Arriving at the size of that initial pool, and deciding whether group testing is even a viable option, depends on multiple factors. Above all, Bilder said, a clinic has to account for the dilution effect — the fact that mixing samples can lower the likelihood of identifying positive cases, potentially resulting in false negatives. The smaller the pool, the less likely that is to happen.
After confirming that a pool is small enough to avoid the dilution effect, there’s still the matter of estimating what percentage of a population is infected. A lower infection rate generally allows for larger, more efficient pools, whereas a higher infection rate will limit pool sizes.
“The way to think about that is: Imagine if you had a positive rate of 50%,” Bilder said. “If you start putting a lot of samples together, basically every group is going to be positive, and then you lose the benefits of group testing.”

In testing individual samples for weeks before it shifted to group testing, the Nebraska Public Health Laboratory concluded that about 5% of the samples it was receiving were positive for the novel coronavirus. As testing ramps up, that percentage will likely drop if the public continues to practice social distancing, which should only improve the efficiency of group testing. As the positive rate falls from 5% to 2%, Bilder said, the increase in testing capacity should rise from the aforementioned 137% to about 264%, on average.
But answering the critical question — Exactly how large should the initial pool be in order to minimize the number of tests? — is tricky, Bilder said. If one large pool comes back negative, a clinic will save more tests than if it had first split those samples into several smaller pools. When a large pool turns up positive, though, a clinic may wind up spending more subsequent tests on ferreting out the infected samples than if it had started smaller.

Now and in the near future, the most common form of group testing — the hierarchical testing used by the Nebraska Public Health Laboratory — is also the one best suited to minimize tests and maximize the people being tested. When infection rates drop substantially lower, though, alternative forms could prove even more efficient, Bilder said.
One alternative, known as array testing, arranges individual samples into a Battleship-like grid of rows and columns. In a 10-by-10 grid of 100 samples, a lab would group together portions of every sample from a respective row or column, then test the 20 resulting pools. If a sample’s row and column both tested positive, the remainder of that sample would be individually tested. In the simplest implementation, all other samples would be declared negative.
“As we continue to increase the amount of testing that’s done in the United States, we can start thinking about having a lower disease prevalence among those people being tested, because we’re including individuals without symptoms,” Bilder said. “And that’s when going to some of these other methods could be beneficial.”
Because the team’s app caters to both hierarchical and array testing, users can easily compare the results of each to determine which would be more efficient. Bilder said that functionality and the app’s overall ease of use owe much to the programming prowess of Hitt, who will graduate with her doctorate in May.
As part of her dissertation, Hitt actually built an entire package in the R programming language that expands on a prior one dedicated to group testing. When users click the “Calculate” button, the app calls in mathematical functions from the new package to perform the necessary calculations and generate results. To ease users into the app and give them a sense of how changes to certain variables can alter those results, Hitt also coded an “Example” button that populates all of the fields with random but reasonable values.

Bilder’s longtime conversations with colleagues at the Nebraska Public Health Laboratory, the Association of Public Health Laboratories, and other sites that employ group testing ultimately informed the app’s design, Hitt said.
“It’s been important for us to get their feedback throughout this process and just improve it as much as we can,” she said. “We used that information to come up with a more user-friendly app.
“We realize that the people who probably benefit most from its functions are people who don’t have a math-stat background, or if they do, it’s not extensive. And they may not have any programming experience. So it was important to us to create this app to reach those people. Asking easy-to-understand questions that elicit the information we need, providing easy fill-in boxes and sliders and radio buttons — it doesn’t require any coding (for users), and that was the goal.”
Accounting for other considerations, including some that Bilder and fellow statisticians have spent years studying, could optimize group testing even further. Contextual information — in the case of COVID-19, whether someone is showing symptoms or has been in contact with infected people — could help slot samples into low- and high-risk pools, better calibrating the initial pool size and form of group testing.
The researchers have also looked at the optimal group-testing strategies when testing for more than one disease at a time, as many labs do when screening for sexually transmitted diseases. Applying that approach to the novel coronavirus, Bilder said, could accelerate the process of distinguishing it from other viruses that produce similar symptoms.
“Imagine if we could test for both the flu and COVID-19 at exactly the same time,” he said.
A recent personal scare only reinforced Bilder’s belief that group testing should be implemented on a far wider scale. Just a couple of weeks before the Nebraska Public Health Laboratory reached out to him for guidance, Bilder came down with COVID-19-like symptoms. Like so many before and after him, though, he didn’t meet the strict testing requirements — an issue that stems in large part from shortages that group testing could help address.
“If there was ever a time for group testing, now’s the time,” he said. “It’s not going to completely solve the testing problem, but it is an important component of the overall solution to the problem.”