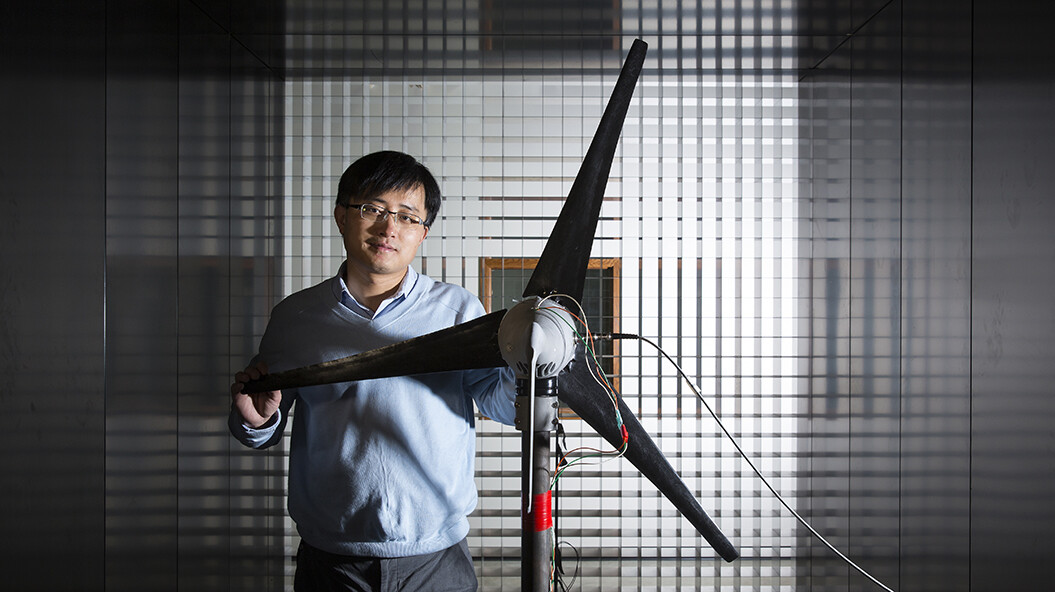
Electrical signals regularly provide vital information on the condition of patients, with cardiologists using EKGs to detect heart arrhythmias and neurologists employing EEGs to pinpoint brain injuries.
With a new $1.5 million award from the U.S. Department of Energy, UNL electrical engineers are teaming with General Electric Global Research to develop a health monitoring system that applies the same principle toward diagnosing and prognosticating damage in wind turbines.
Mechanical problems with blades, bearings, gears and other turbine components often produce vibrations that modify the electrical signals produced by their generators, according to the UNL team. The researchers are developing an online, real-time monitoring system that automatically analyzes the frequencies of these signals, thereby allowing it to distinguish and classify signatures associated with common mechanical flaws.
“If there’s some failure in the turbine, we’ll be able to detect it, identify its location and (nature), and ultimately determine the root cause of it,” said project leader Wei Qiao, associate professor of electrical and computer engineering. “The failure may stem from minor damage or a small defect, but our technology will be able to detect this fault at an early stage.”
The system will also leverage years of historical data to offer a prognosis for any detected flaws, performing probability analyses of how damage will progress and spread over time. These projections will allow the system to predict the functional lifespan of both damaged components and the turbine as a whole, said Qiao.
Unlike traditional monitoring systems, the UNL team’s design will not require the installation of sensors or data-acquisition devices. Instead, Qiao said, it will be capable of relying solely on the electrical signals that are produced at generator terminals and readily available in a turbine control system. As a result, the new system could easily replace or even interface with existing ones.
The team expects its monitoring system to reduce both the maintenance costs and operational downtime of wind turbines by 40 percent. Qiao cited such progress as especially important for a technology that bears the brunt of the very environments it’s intended to help protect.
“Wind energy has been developing rapidly for about two decades, but there are still challenges to using it,” Qiao said. “One of those challenges is reliability. Because wind turbines are installed in harsh outdoor environments – subject to storms, ice, everything – their failure rate is higher than that of counterparts in traditional power plants.”
Qiao said this failure rate is largely to blame for the fact that operational and maintenance expenses constitute 10 to 15 percent of lifetime costs in onshore turbines and 20 to 35 percent in offshore units.
In illustrating the potential domino effect of even a small defect, Qiao said a turbine’s bearing might initially continue to operate after minor damage. Yet the vibrations produced by this malfunction could gradually warp a drivetrain or damage other components, eventually reaching a critical mass that destroys an entire gearbox or generator.
Despite this, inspections and maintenance in the wind industry have typically transpired according to routine schedules rather than the actual condition of turbines, Qiao said. Consequently, repairs often ensue only after turbines have stopped functioning – and after potential financial and time savings have already been squandered.
“When a defect or fault occurs in a big mechanical system like a wind turbine, the system has to stop operating for a long time – days, months, even longer,” Qiao said. “By monitoring the health condition of the wind turbine, we can schedule maintenance and prepare resources more intelligently. This will be very valuable for the wind industry.”
Trials of error
Though mechanical faults produce vibrations that represent the key to diagnosing damage, a turbine’s standard activity produces vibrations of its own. To sort fault-relevant signals from irrelevant noise, the team will develop a high-resolution signal-processing method that effectively increases the ratio between the two.
However, Qiao said extracting a signal is further complicated by the fact that the rotational speeds of turbine rotors and shafts vary with shifts in the wind. This variation diminishes the usefulness of conventional frequency analysis methods by forcing the system to constantly account for changes in standard vibrations.
In response, the team’s system will continuously process signals in a way that essentially converts fluctuating vibration-related information into a constant form. Doing so will permit the use of conventional analysis methods for identifying vibrations that can be attributed to faults in the turbine, according to Qiao.
Over the next year, the engineering team will collaborate with General Electric to field-test its monitoring system in GE-produced turbines across the United States. GE is the country’s largest manufacturer of commercial wind turbines, Qiao said, having produced more than 25,000 to date.
The researchers also will evaluate the system’s performance in a handful of rural Nebraska turbines that were installed as part of the Department of Energy’s Wind for Schools Program. The team will further assess its design using several miniature turbines and a wind tunnel located at the Qiao-directed Power and Energy Systems Laboratory on the UNL campus.
Qiao’s UNL collaborators on the project include Liyan Qu, assistant professor of electrical and computer engineering, and Jerry Hudgins, professor and chair of electrical and computer engineering.