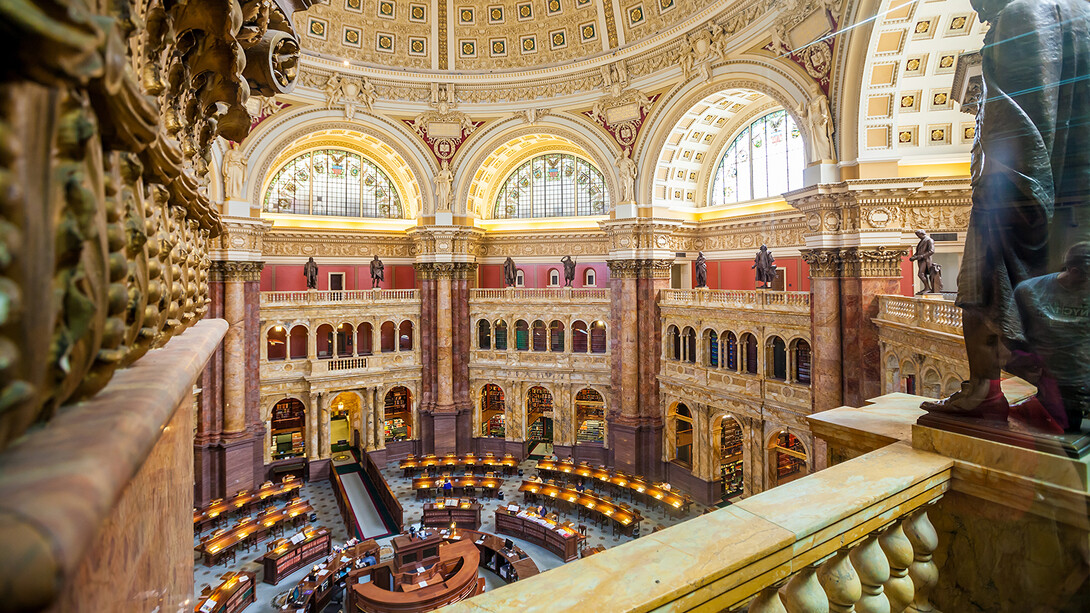
How can the United States Library of Congress — one of the world’s largest repositories of information — bring its collections into the digital age?
It’s a question library leadership has been attempting to answer, and a collaboration between the Library of Congress and University of Nebraska–Lincoln scholars and students has laid a strong foundation for machine learning to play a role in future digital strategies.
In 2018, the Digital Strategy Division of the Library of Congress released a five-year digital strategy for the library, with a goal of maximizing the value of its collections for research. As part of that strategy, the library began seeking a collaboration to test machine learning across different materials, since the library’s collections are so varied.
The Aida digital libraries research lab, led by Husker researchers Elizabeth Lorang, associate professor in University Libraries, and Leen-Kiat Soh, professor of computer science and engineering, were awarded a research services contract following a call for proposals from the library.
Aida is centered on making cultural heritage materials that have been digitized more accessible through computational image analysis tools and machine learning. The team has received grant funding from the National Endowment for the Humanities and the Institute of Museum and Library Services, among others.
“We’re interested in some of these same questions that the Library of Congress was asking,” Lorang said. “What can we do with the material that’s being made available; and how do we find things? We’re also looking forward to how can we have an impact from the moment digital libraries are being developed so that we can maximize the process from the beginning all the way to the end.”
To complete the work for the library, Chulwoo Pack and Yi Liu, both doctoral students in computer science and engineering, spent six weeks in the Library of Congress in summer 2019, exploring and investigating various image-processing and machine-learning techniques on a variety of archival materials. From Lincoln, Lorang and Soh served as senior advisers for the project. The team continued its work for another six weeks during the fall semester and delivered preliminary results to the Library of Congress Nov. 6.
“We didn’t have any very straightforward requirements,” Pack said. “We worked with a supervisor who gave us new ways to think about things, and we generated a list of ideas of documents and metadata we could explore.”
Among the explorations of machine learning that Liu and Pack experimented with was enriching metadata by segmenting and cataloging types of visual components. For example, a newspaper page might have chunks of words along with a picture, cartoon and an advertisement. Pack and Liu experimented with ways computer programming could make those determinations and simultaneously add that data to the image’s digital file.
“Another project was on differentiation between handwritten material and printed, or typed material,” Liu said. “(In machine learning), this would tell if further processes would be needed for classification or segmentation, based on the type of content.”
Soh and Lorang said the completed research was illuminating for both the Nebraska and Library of Congress teams, and that the overall project was a success.
“I think this really informed how the practice of machine learning could be incorporated into their processes,” Soh said. “Before, the thought of machine learning was a bit mysterious, but through this collaboration, there is now a tangible, viable approach. They’ve seen how it can work in a methodical way, and as a result, more informed about how to consider the factors and parameters involved in machine learning.”
“We’ve heard already from the leads at the Library of Congress how much this has informed and influenced their thinking about the role of machine learning,” Lorang said. “I think they were just blown away by the work from this summer.
“It’s pretty remarkable to think that our research team from the University of Nebraska is helping the Library of Congress, and we were the experts to help them consider machine learning and the overall strategy around it.”
The team will travel to the Library of Congress in January to present a full suite of deliverables, including code and documentation, curated data sets and a written report.